Top 5 Natural Language Processing (NLP) Libraries for 2024
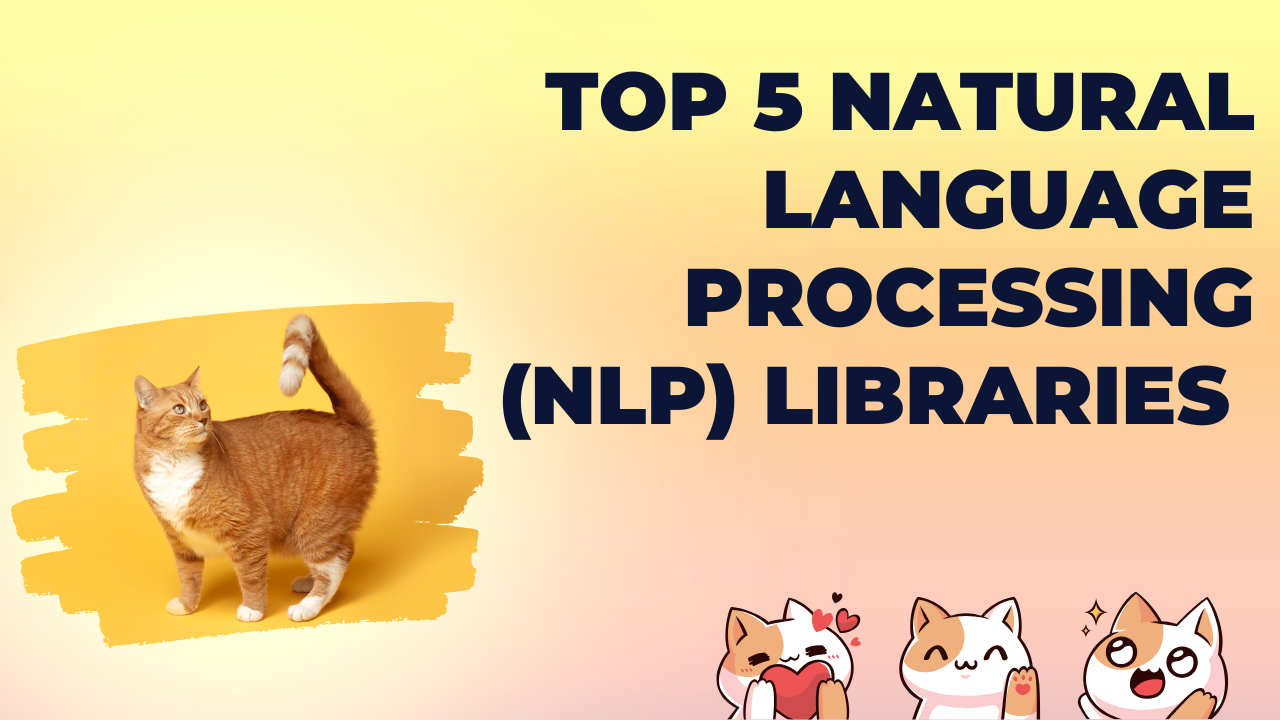
In the dynamic field of data science, Natural Language Processing (NLP) continues to play a pivotal role in enabling machines to understand and interpret human language. As we move into 2024, the demand for advanced NLP applications—from chatbots and sentiment analysis to machine translation and text summarization—has never been higher. To meet these evolving needs, numerous NLP libraries have emerged, each offering unique features and capabilities. This blog post explores the top 5 NLP libraries every data scientist should consider mastering in 2024, detailing their strengths, use cases, and providing affiliate links to resources that can help you get started.
1. NLTK (Natural Language Toolkit)
Overview
NLTK is one of the most widely used open-source libraries for NLP in Python. It provides easy-to-use interfaces to over 50 corpora and lexical resources, along with a suite of text processing libraries for classification, tokenization, stemming, tagging, parsing, and semantic reasoning.
Features
- Comprehensive suite of text processing libraries
- Extensive documentation and tutorials
- Support for various linguistic data formats
- Integration with other Python libraries like NumPy and SciPy
Benefits
- Ideal for educational purposes and beginners
- Facilitates rapid prototyping of NLP applications
- Large community support ensuring continuous updates and improvements
Use Cases
- Text classification and sentiment analysis
- Tokenization and text preprocessing
- Building educational tools and NLP tutorials
[Download NLTK](#)
2. spaCy
Overview
spaCy is an open-source library designed for advanced NLP in Python. Unlike NLTK, spaCy focuses on providing a fast and efficient pipeline for processing large volumes of text, making it suitable for real-world production environments.
Features
- High-performance NLP pipeline
- Pre-trained models for multiple languages
- Seamless integration with deep learning frameworks like TensorFlow and PyTorch
- Robust support for named entity recognition, part-of-speech tagging, and dependency parsing
Benefits
- Optimized for speed and efficiency, handling large datasets with ease
- Simplifies the development of production-ready NLP applications
- Active development ensuring up-to-date features and improvements
Use Cases
- Large-scale text processing and analysis
- Building chatbots and virtual assistants
- Enhancing search engine capabilities with better understanding of queries
[Explore spaCy](#)
3. TensorFlow
Overview
TensorFlow, developed by Google, is a versatile open-source library primarily used for machine learning and deep learning applications. Its extensive support for NLP tasks makes it a powerful tool for developing sophisticated language models.
Features
- Comprehensive ecosystem with tools like TensorFlow Hub and TensorFlow Extended
- Support for building custom neural network architectures
- Integration with Keras for simplified model building
- GPU acceleration for faster training processes
Benefits
- Highly scalable, suitable for both research and production
- Extensive community and resources for support and development
- Facilitates the creation of state-of-the-art NLP models like BERT and GPT
Use Cases
- Developing deep learning-based language models
- Machine translation and language generation
- Sentiment analysis and text summarization using neural networks
[Start with TensorFlow](#)
4. PyTorch
Overview
PyTorch, developed by Facebook's AI Research lab, is an open-source library renowned for its flexibility and ease of use in building deep learning models. Its dynamic computation graph makes it particularly attractive for research and development in NLP.
Features
- Dynamic computation graph for flexibility in model development
- Strong support for GPU acceleration
- Extensive library of pre-trained models and tools
- Seamless integration with Python, making it easy to learn and implement
Benefits
- Ideal for research and experimentation due to its flexible architecture
- Active community contributing to a rich ecosystem of tools and extensions
- Simplifies the development of complex NLP models with ease
Use Cases
- Researching and developing new NLP algorithms
- Building and training custom language models
- Implementing reinforcement learning in language-based applications
[Explore PyTorch](#)
5. Hugging Face Transformers
Overview
Hugging Face Transformers is an open-source library that provides a wide range of pre-trained transformer models, including BERT, GPT-3, and T5. It has become the go-to library for many practitioners looking to implement cutting-edge NLP solutions with minimal effort.
Features
- Access to a vast repository of pre-trained transformer models
- Easy-to-use APIs for fine-tuning models on specific tasks
- Integration with both TensorFlow and PyTorch
- Support for numerous languages and specialized domains
Benefits
- Accelerates the development process by leveraging pre-trained models
- Reduces the need for extensive computational resources for training from scratch
- Continuously updated with the latest advancements in transformer-based models
Use Cases
- Text generation and completion
- Question answering systems
- Advanced sentiment analysis and emotion detection
[Get Started with Hugging Face](#)
Recommended Books and Courses
Books
1. "Natural Language Processing with Python" by Steven Bird, Ewan Klein, and Edward Loper
A foundational text for understanding NLP concepts using Python and NLTK. [Buy on Amazon]
2. "Deep Learning for Natural Language Processing" by Palash Goyal, Sumit Pandey, and Karan Jain
An in-depth guide to applying deep learning techniques to NLP tasks. [Buy on Amazon]
Courses
1. [Natural Language Processing with Deep Learning](#) on Coursera
Learn how to build and deploy advanced NLP models using deep learning techniques.
Conclusion
As NLP continues to evolve, selecting the right library can significantly impact the efficiency and effectiveness of your projects. Whether you're a beginner starting with NLTK or spaCy, or an experienced data scientist leveraging the power of TensorFlow, PyTorch, or Hugging Face Transformers, mastering these tools will empower you to build robust and intelligent language applications.
Investing time in learning these libraries will not only enhance your technical skills but also open doors to exciting opportunities in the realm of natural language processing. Additionally, leveraging recommended books and courses can provide structured learning paths to accelerate your proficiency in NLP.
Start your journey towards mastering the top NLP libraries of 2024 and stay ahead in the rapidly advancing field of data science and machine learning.
---
*Disclaimer: This post contains affiliate links, meaning we may earn a commission if you click a link and make a purchase at no additional cost to you. We only recommend products and services we believe will add value to our readers.*
Comments
Please log in to leave a comment.
No comments yet.