Top 10 Data Science Tools Every Analyst Should Master in 2025
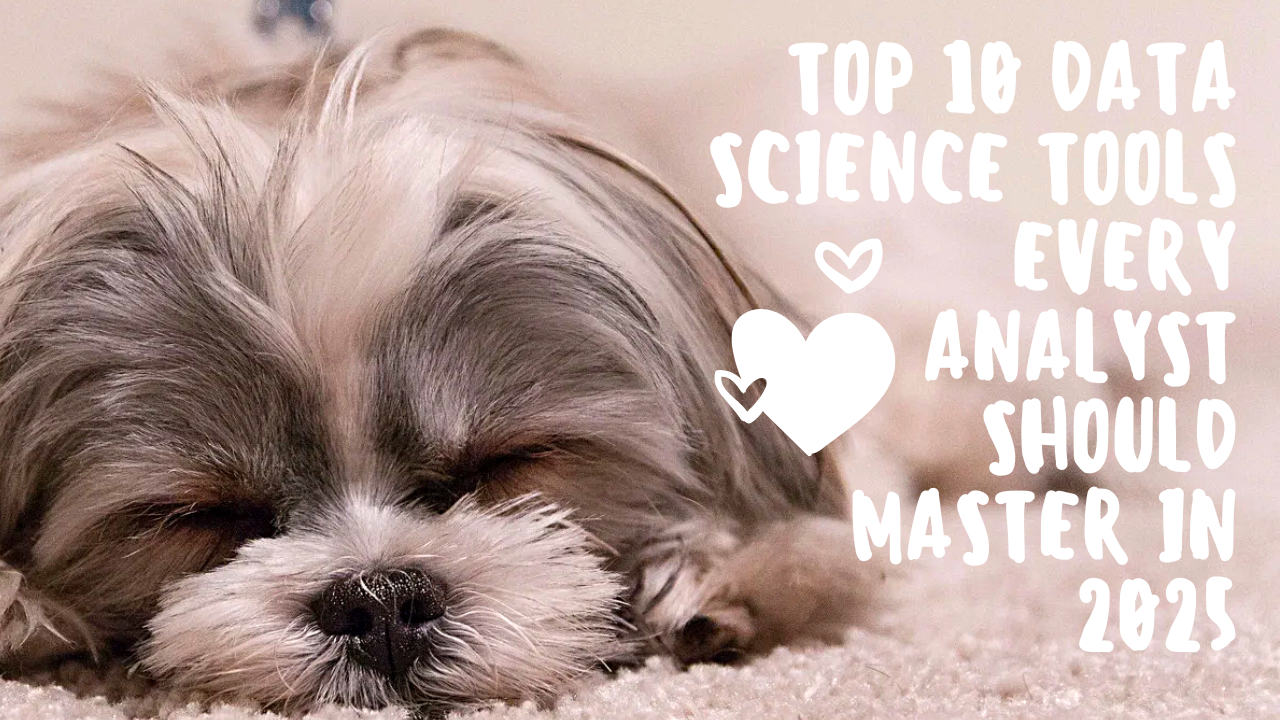
In the rapidly evolving field of data science, staying updated with the latest tools and technologies is crucial for analysts aiming to deliver insightful and impactful results. Whether you're a beginner embarking on your data journey or a seasoned analyst seeking to enhance your toolkit, mastering the right tools can significantly boost your productivity and the quality of your analyses. In this blog post, we explore the top 10 data science tools every analyst should master in 2024, highlighting their features, benefits, and use cases. Additionally, we've included affiliate links to some of these tools and recommended courses to help you get started.
---
## 1. Tableau (Data Visualization)
### Overview
Tableau is a leading data visualization tool that transforms raw data into actionable insights through interactive and shareable dashboards. Its intuitive drag-and-drop interface makes it accessible to users with varying levels of technical expertise.
### Features
- Interactive Dashboards: Create dynamic and interactive visualizations.
- Data Connectivity: Connects to various data sources, including SQL databases, spreadsheets, and cloud services.
- Real-Time Data Analysis: Analyze data in real-time for timely decision-making.
### Benefits
- User-Friendly Interface: Minimal learning curve compared to other visualization tools.
- Robust Community Support: Extensive resources and community forums for assistance.
- Scalability: Suitable for both small businesses and large enterprises.
### Use Cases
- Business Reporting: Generate comprehensive business reports with visual representations.
- KPI Tracking: Monitor key performance indicators in real-time.
- Market Analysis: Analyze market trends and consumer behavior patterns.
[Get Tableau Now]
---
## 2. Looker (Business Intelligence)
### Overview
Looker is a powerful business intelligence platform that facilitates data exploration and discovery. It offers a modern approach to data analysis by leveraging the LookML language for data modeling.
### Features
- Data Modeling with LookML: Customizable data models tailored to specific business needs.
- Embedded Analytics: Integrate analytics into websites and applications.
- Collaborative Tools: Share insights and collaborate with team members seamlessly.
### Benefits
- Customizable Dashboards: Tailor dashboards to specific user roles and requirements.
- Seamless Integration: Integrates well with various data warehouses and tools.
- Enhanced Data Security: Robust security features to protect sensitive data.
### Use Cases
- Sales Analytics: Track sales performance and identify growth opportunities.
- Customer Insights: Gain a deeper understanding of customer behaviors and preferences.
- Operational Efficiency: Monitor and improve business processes.
[Explore Looker](#)
---
## 4. AWS (Cloud Services)
### Overview
Amazon Web Services (AWS) is a comprehensive cloud platform offering a wide range of services, including computing power, storage, and databases, essential for data science projects.
### Features
- Scalable Infrastructure: Easily scale resources up or down based on demand.
- Big Data Solutions: Services like Amazon EMR and Redshift for big data processing.
- Machine Learning Services: Tools like SageMaker for building, training, and deploying ML models.
### Benefits
- Reliability: High availability and reliability with global data centers.
- Security: Advanced security features to protect data.
- Cost-Effective: Pay-as-you-go pricing model reduces upfront costs.
### Use Cases
- Data Storage and Management: Store and manage large datasets efficiently.
- Machine Learning: Develop and deploy machine learning models at scale.
- Data Processing: Handle big data processing tasks with ease.
[Explore AWS](#)
---
## 5. Google Cloud Platform (Data Services)
### Overview
Google Cloud Platform (GCP) offers a suite of cloud computing services that provide robust infrastructure and machine learning capabilities, making it a preferred choice for data scientists and analysts.
### Features
- BigQuery: Fully-managed data warehouse for large-scale data analysis.
- AI and Machine Learning: Advanced AI tools and pre-trained models.
- Data Integration: Seamlessly integrate with various data sources and services.
### Benefits
- High Performance: Optimized for speed and efficiency in data processing.
- Innovative Tools: Access to cutting-edge machine learning and AI technologies.
- Global Infrastructure: Leverage Google’s global network for reliable performance.
### Use Cases
- Real-Time Analytics: Perform real-time data analysis and reporting.
- Predictive Modeling: Develop predictive models to forecast trends.
- Data Warehousing: Efficiently store and query large datasets.
[Get Started with GCP](#)
---
## 6. Python (Programming Language)
### Overview
Python is a versatile and widely-used programming language in data science, known for its simplicity and extensive library support.
### Features
- Extensive Libraries: Pandas, NumPy, Scikit-learn, and TensorFlow.
- Readable Syntax: Easy to learn and write, making it ideal for beginners.
- Community Support: Large and active community contributing to continuous improvements.
### Benefits
- Flexibility: Suitable for a wide range of applications, from data analysis to machine learning.
- Integration Capabilities: Easily integrates with other tools and platforms.
- Scalability: Can handle projects of varying sizes and complexities.
### Use Cases
- Data Cleaning and Preparation: Efficiently preprocess and clean datasets.
- Machine Learning: Develop and train machine learning models.
- Automation: Automate repetitive data tasks and workflows.
[Learn Python for Data Science](#)
---
## 7. R (Programming Language)
### Overview
R is a statistical programming language designed for data analysis and visualization, favored by statisticians and data miners.
### Features
- Statistical Analysis: Robust support for various statistical techniques.
- Visualization Tools: Libraries like ggplot2 are used to create detailed visualizations.
- Data Manipulation: Efficient data manipulation with packages like dplyr and tidyr.
### Benefits
- Specialized for Statistics: Ideal for complex statistical analyses.
- Comprehensive Package Ecosystem: Thousands of packages available for diverse data science tasks.
- Strong Community: An active community that provides support and continuous enhancement.
### Use Cases
- Exploratory Data Analysis: Perform in-depth analysis to uncover insights.
- Statistical Modeling: Develop and validate statistical models.
- Reporting and Visualization: Create detailed reports and visual representations of data.
[Master R for Data Science](#)
---
## 8. Jupyter Notebooks
### Overview
Jupyter Notebooks are an open-source web application that allows you to create and share documents containing live code, equations, visualizations, and narrative text.
### Features
- Interactive Coding Environment: Write and execute code in real-time.
- Support for Multiple Languages: Primarily supports Python, R, and Julia.
- Integration with Data Sources: Connect to various databases and APIs for data retrieval.
### Benefits
- Enhanced Collaboration: Share notebooks with team members for collaborative analysis.
- Reproducible Research: Maintain a record of your analysis steps and results.
- Flexible Visualization: Embed interactive visualizations directly in your notebooks.
### Use Cases
- Data Exploration: Explore and visualize data interactively.
- Machine Learning Prototyping: Develop and test machine learning models.
- Educational Purposes: Use as a teaching tool for data science concepts.
[Get Jupyter Notebooks](#)
---
## 9. SQL (Structured Query Language)
### Overview
SQL is the standard language for managing and manipulating relational databases. Proficiency in SQL is essential for querying and managing large datasets effectively.
### Features
- Data Retrieval: Efficiently query databases to retrieve specific data.
- Data Manipulation: Insert, update, and delete records in databases.
- Database Management: Create and manage database structures.
### Benefits
- Universal Use: Widely used across various database systems like MySQL, PostgreSQL, and SQL Server.
- High Performance: Optimized for handling large volumes of data.
- Essential Skill: Fundamental for any data analyst or scientist.
### Use Cases
- Data Analysis: Extract and analyze data for business insights.
- Database Administration: Manage and maintain database systems.
- Reporting: Generate reports based on queried data.
[Learn SQL for Data Analysis](#)
---
## 10. GitHub (Version Control)
### Overview
GitHub is a version control and collaboration platform that allows multiple users to work on projects simultaneously. It's an indispensable tool for managing codebases and ensuring code quality.
### Features
- Version Control: Track changes and manage different versions of your code.
- Collaboration Tools: Facilitate teamwork through features like pull requests and code reviews.
- Integration with CI/CD: Integrate seamlessly with continuous integration and deployment pipelines.
### Benefits
- Improved Collaboration: Streamline teamwork and code sharing among team members.
- Code Management: Maintain a clean and organized codebase.
- Community and Resources: Access a vast repository of open-source projects and resources.
### Use Cases
- Project Management: Manage and track progress on data science projects.
- Code Collaboration: Collaborate with other data scientists and developers.
- Open-Source Contribution: Contribute to and leverage open-source data science tools.
[Join GitHub](#)
## Recommended Books and Courses
### Books
1. "Python for Data Analysis" by Wes McKinney
An essential guide for anyone looking to learn data manipulation and analysis using Python. [Buy on Amazon](#)
2. "R for Data Science" by Hadley Wickham & Garrett Grolemund
A comprehensive resource for mastering data science with R. [Buy on Amazon]
### Courses
1. [Microsoft Power BI - A Complete Introduction](#) on Coursera
Learn how to create impactful visualizations and dashboards with Power BI.
---
## Conclusion
Mastering the right data science tools is pivotal for analysts aiming to excel in their careers and deliver meaningful insights. The tools listed above—ranging from data visualization platforms like Tableau and Power BI to programming languages like Python and R and cloud services like AWS and Google Cloud—cover a broad spectrum of functionalities essential for today's data-driven landscape.
Investing time in learning and mastering these tools will enhance your analytical capabilities and open up new opportunities in the ever-expanding field of data science. Additionally, leveraging resources like books and online courses can provide structured learning paths to accelerate your proficiency.
Remember, the key to effective data analysis lies in your tools and how you apply them to solve real-world problems. Choose the tools that best fit your project requirements and workflow, and continue to adapt as new technologies emerge.
Start your journey to becoming a data science expert today by exploring these essential tools!
---
*Disclaimer: This post contains affiliate links, meaning we may earn a commission if you click a link and make a purchase at no additional cost. We only recommend products and services we believe will add value to our readers.*
Comments
Please log in to leave a comment.
No comments yet.