Preparing for a Data Engineer Interview 2025
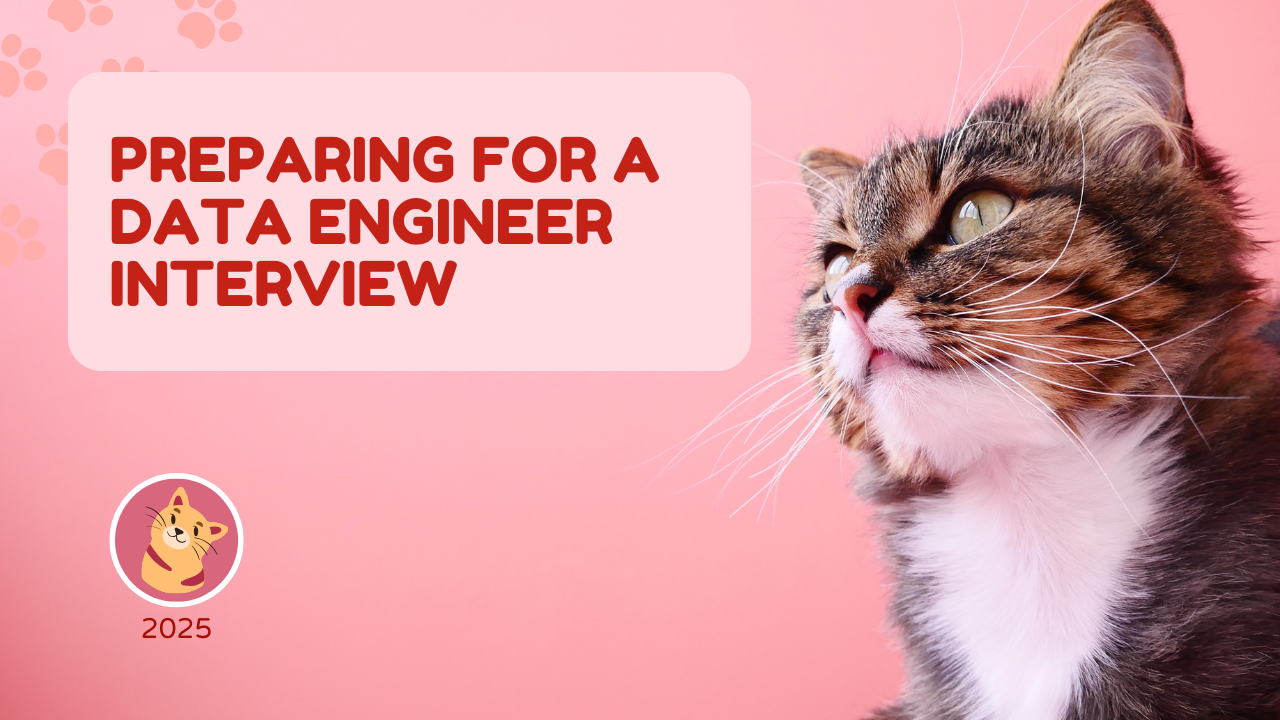
Data engineering is at the heart of modern analytics, bridging raw data and actionable insights. If you're gearing up for a data engineer interview, having a strategic preparation plan that covers both technical skills and practical experience is crucial. Drawing from industry experience, this guide will help you confidently navigate the interview process.
1. Understand the Role and Responsibilities
Key Areas to Focus On:
-
Data Quality Assessment and Model Training Workflows:
- Learn how to evaluate datasets for missing values, biases, and scaling problems.
- Understand the steps involved in preparing data for machine learning models.
- Familiarize yourself with data preprocessing techniques to improve model performance.
-
Automation with Python and Bash:
- Gain proficiency in scripting to automate repetitive tasks.
- Learn error handling to create robust and reliable scripts.
- Explore how automation enhances efficiency in data pipelines.
-
Open-Source Model Training Workflows:
- Study popular tools and frameworks like scikit-learn, TensorFlow, and PyTorch.
- Understand how to process raw data and feed it into machine learning models.
- Review end-to-end workflows from data ingestion to model deployment.
2. Technical Skills Preparation
Python:
-
Data Manipulation:
- Master libraries like Pandas and NumPy are used for data analysis.
- Practice data cleaning, transformation, and aggregation techniques.
-
Automation and Error Handling:
- Use modules such as os, sys, and subprocess for system-level operations.
- Implement try-except blocks to handle exceptions gracefully.
Google Colab and Sheets:
- Integration:
- Learn to use APIs or libraries like gspread to interact with Google Sheets.
- Automate data extraction and updates between Colab notebooks and Sheets.
Bash Scripting:
- Shell Commands:
- Familiarize yourself with command-line operations, file manipulations, and process management.
- Script Writing:
- Write reusable scripts to streamline tasks like data transfers and environment setups.
GitHub and Data Formats:
- Version Control:
- Understand Git basics: cloning repositories, committing changes, branching, and merging.
- Data Handling:
- Work with various data formats (CSV, JSON, Parquet) and understand their use cases.
Real-World Insight:
While working as a Data Scientist, I frequently collaborated on GitHub repositories to manage codebases for data processing and model training. Handling data stored in different formats required flexibility and a solid understanding of data serialization and deserialization methods.
3. Practical Hands-On Experience
Sample Project Setup:
-
Data Ingestion:
import pandas as pd # Read data from a CSV file df_csv = pd.read_csv('data.csv') # Fetch data from an API import requests response = requests.get('https://api.example.com/data') df_api = pd.DataFrame(response.json())
-
Quality Checks:
# Check for duplicates duplicates = df_csv[df_csv.duplicated()] # Identify missing values missing_values = df_csv.isnull().sum()
-
Automation Scripts:
Python Script Example:
import subprocess # Run a Bash script from Python subprocess.call(['sh', './script.sh'])
Bash Script Example (script.sh):
#!/bin/bash # Simple Bash script to backup data cp data.csv backup/data_$(date +%F).csv
-
Model Training:
from sklearn.model_selection import train_test_split from sklearn.ensemble import RandomForestClassifier # Split data X = df_csv.drop('target', axis=1) y = df_csv['target'] X_train, X_test, y_train, y_test = train_test_split(X, y, test_size=0.2) # Train model model = RandomForestClassifier() model.fit(X_train, y_train)
-
Reporting Findings:
- Use visualization libraries like Matplotlib or Seaborn to create charts and graphs.
- Share results through dashboards or export to Google Sheets for stakeholders.
Real-World Insight:
I designed and executed sophisticated marketing mix strategies at a media and marketing firm, including A/B testing and multi-touch attribution models. Automating data collection and processing improved efficiency and enhanced the accuracy of client insights.
4. Anticipate Interview Questions
Technical Questions:
-
Handling Missing or Corrupted Data:
Answer:
- Identify Missing Data: Use df.isnull().sum() to find columns with missing values.
- Decide on Strategy: Depending on the data and context:
- Remove rows/columns with excessive missing data.
- Impute missing values using mean, median, mode, or predictive models.
- Use advanced techniques like K-Nearest Neighbors imputation.
-
Python Script to Identify Duplicate Rows:
duplicates = df[df.duplicated()] print("Duplicate Rows:") print(duplicates)
-
Training an Open-Source Model Using Data from a Google Sheet:
Answer:
-
Use gspread library to read data:
import gspread from oauth2client.service_account import ServiceAccountCredentials scope = ["https://spreadsheets.google.com/feeds",'https://www.googleapis.com/auth/drive'] creds = ServiceAccountCredentials.from_json_keyfile_name('client_secret.json', scope) client = gspread.authorize(creds) sheet = client.open('DataSheet').sheet1 data = sheet.get_all_records() df = pd.DataFrame(data)
-
Proceed with preprocessing and model training as usual.
-
Behavioral Questions:
-
Automating a Data Processing Workflow:
Example Answer:
In my previous role, I automated the data ingestion and cleaning process for daily sales data. By scripting the ETL pipeline using Python and scheduling it with cron jobs, we reduced manual effort by 80%. We minimized errors, allowing the team to focus on data analysis rather than data preparation.
-
Solving a Critical Data Quality Issue:
Example Answer:
After noticing inconsistent metrics, I discovered that data from one source was misaligned due to a timezone mismatch. I implemented a data validation step that checked for consistency in timestamp formats and adjusted them accordingly. This resolved discrepancies and improved the reliability of our analytics reports.
-
Case Study Approach:
When given a large dataset with human-labeled data:
- Step 1: Perform exploratory data analysis to understand the dataset's structure.
- Step 2: Check for missing values, duplicates, and inconsistent entries.
- Step 3: Validate labels by spot-checking samples or using statistical methods to detect anomalies.
- Step 4: Clean the data using appropriate techniques.
- Step 5: Document the quality assessment and cleaning steps for transparency.
5. Understand the Interview Process
-
Technical Assessments:
- Coding exercises in Python or SQL.
- Debugging a script or fixing a data pipeline.
- Designing a data model or schema based on given requirements.
-
Data Quality Impact Discussions:
- Be prepared to explain how poor data quality can lead to biased models or incorrect business insights.
- Discuss methods to ensure data integrity throughout the data lifecycle.
-
Collaboration Scenarios:
- Share experiences of working in cross-functional teams.
- Highlight your communication skills and ability to translate technical concepts for non-technical stakeholders.
Real-World Insight:
Collaborating with senior data engineers and AI modeling teams requires clear communication and a shared understanding of project goals. In my roles, regular meetings and updates ensured alignment, and using collaborative tools like Git and shared documentation facilitated smooth teamwork.
6. Prepare Questions for the Interviewers
-
Tools and Frameworks:
- Question: What are your team's primary tools and technologies for data engineering tasks?
-
Data Quality and Model Performance:
- Question: How does the team approach iterative improvements in data quality and refining machine learning models?
-
Challenges in Processing Human Data:
- Question: What are your biggest challenges when handling human-labeled data, and how does the team address them?
7. Logistics and Team Dynamics
-
Office Attendance:
- Be honest about your availability.
- Express willingness to attend important meetings or collaborative sessions in person if required.
-
Team Collaboration:
- Emphasize adaptability in working with diverse teams.
- Highlight any experience with remote collaboration tools.
Real-World Insight:
In previous roles, balancing remote work with periodic office visits was essential for maintaining team cohesion. Utilizing communication platforms and scheduling regular check-ins helped bridge any gaps.
Conclusion
Preparing for a data engineer interview involves sharpening technical skills, gaining practical experience, and understanding the role's nuances. Following this structured plan and drawing on real-world examples, you'll be well-equipped to navigate the interview confidently.
Remember:
-
Demonstrate Problem-Solving Abilities:
- Show how you approach challenges methodically.
- Provide examples of innovative solutions you've implemented.
-
Showcase Practical Experience:
- Highlight projects and experiences that align with the role.
- Discuss the impact of your work on previous organizations.
-
Communicate Effectively:
- Articulate your thoughts clearly.
- Be prepared to explain complex concepts in simple terms.
Additional Resources
-
Books:
- Data Engineering with Python by Paul Crickard
- Designing Data-Intensive Applications by Martin Kleppmann
-
Practice Platforms:
- LeetCode for coding practice
- HackerRank for SQL and data manipulation
Note: This guide is based on collective experiences in data engineering roles across various industries. The insights provided aim to help candidates prepare effectively for data engineer interviews by focusing on critical skills and practical knowledge.
Comments
Please log in to leave a comment.
No comments yet.