Building Scalable Machine Learning Models with Google Cloud Platform
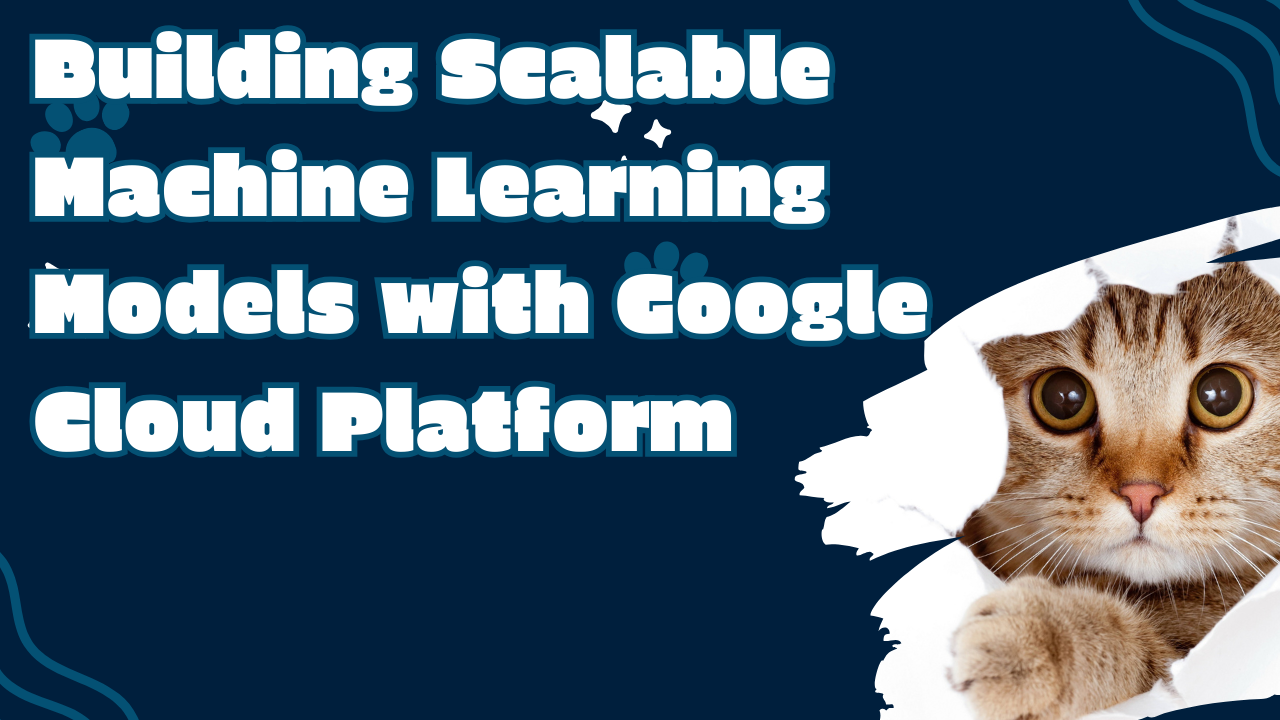
In today's data-driven world, building scalable machine learning (ML) models is essential for organizations that leverage data for strategic decision-making. Google Cloud Platform (GCP) offers powerful tools and services that simplify the large-scale development, deployment, and management of ML models. This guide explores how to harness GCP to build scalable machine learning models, discusses best practices, and provides real-world examples to help you succeed in your ML endeavors.
Table of Contents
1. Introduction to Scalable Machine Learning
2. Why Choose Google Cloud Platform for ML?
3. Key GCP Services for Building Scalable ML Models
- Google AI Platform
- BigQuery
- Google Kubernetes Engine (GKE)
4. Best Practices for Building Scalable ML Models on GCP
5. Real-world examples of Scalable ML Models on GCP
6. Recommended Books and Courses
7. Conclusion
8. Disclaimer
1. Introduction to Scalable Machine Learning
Scalability in machine learning refers to the ability of a system to handle increasing amounts of data and complex computations without compromising performance. As datasets grow larger and models become more sophisticated, ensuring that your ML infrastructure can scale efficiently becomes crucial. Scalable ML models enable organizations to process vast amounts of data, deploy models seamlessly, and achieve faster insights, thereby driving better business outcomes.
2. Why Choose Google Cloud Platform for ML?
Google Cloud Platform stands out as a leading choice for building scalable machine learning models due to its robust infrastructure, advanced AI capabilities, and comprehensive suite of ML tools. Here are some reasons why GCP is ideal for scalable ML:
- Advanced AI and ML Tools: GCP offers cutting-edge tools like TensorFlow and integrated services that streamline ML workflows.
- Scalability and Flexibility: GCP’s infrastructure can scale automatically to handle varying workloads, ensuring optimal performance.
- Integration with Other Services: Seamlessly integrate with other GCP services such as BigQuery for data storage and analysis.
- Security and Compliance: GCP provides robust security features and compliance certifications, ensuring your data and models are protected.
3. Key GCP Services for Building Scalable ML Models
To build and deploy scalable machine learning models on GCP, several key services can be leveraged:
Google AI Platform
Google AI Platform is a managed service that simplifies the process of building, training, and deploying ML models. It supports various frameworks, including TensorFlow, PyTorch, and Scikit-learn, and offers tools for automated machine learning (AutoML).
*Features:*
- Automated Training: Streamline the training process with managed infrastructure.
- Model Deployment: Easily deploy models to production with minimal configuration.
- Integrated Tools: Utilize tools like TensorBoard for monitoring and visualization.
*Benefits:*
- Scalability: Automatically scales resources based on demand.
- Flexibility: Supports multiple ML frameworks and custom containers.
- Ease of Use: User-friendly interface and comprehensive documentation.
[Explore Google AI Platform](https://cloud.google.com/ai-platform)
BigQuery
BigQuery is GCP’s fully managed, serverless data warehouse that enables fast SQL queries using the processing power of Google’s infrastructure. It's optimized for large-scale data analysis and integrates seamlessly with ML tools.
*Features:*
- Real-Time Analytics: Perform real-time data analysis with high-speed querying.
- Machine Learning Integration: Utilize BigQuery ML to build and deploy models directly within BigQuery.
- Data Storage: Handle petabyte-scale datasets effortlessly.
*Benefits:*
- Cost-Effective: Pay only for the storage and processing you use.
- Performance: Achieve high-speed data retrieval and processing.
- Integration: Easily integrate with other GCP services and external tools.
[Discover BigQuery](https://cloud.google.com/bigquery)
Google Kubernetes Engine (GKE)
GKE is a managed Kubernetes service that simplifies containerized applications' deployment, management, and scaling, including ML models.
*Features:*
- Automated Operations: Manage clusters with automated updates and scaling.
- Container Orchestration: Efficiently orchestrate containers for ML workloads.
- Integration with CI/CD: Seamlessly integrate with continuous integration and deployment pipelines.
*Benefits:*
- Scalability: Automatically scale your applications based on demand.
- Flexibility: Deploy ML models in containers for consistent environments.
- Reliability: Benefit from Google’s robust infrastructure and security features.
[Learn More About GKE](https://cloud.google.com/kubernetes-engine)
4. Best Practices for Building Scalable ML Models on GCP
Building scalable ML models on GCP requires adherence to best practices to ensure efficiency, reliability, and performance:
- Optimize Data Storage: Utilize BigQuery to handle large datasets and ensure well-organized data for efficient querying and processing.
- Leverage AutoML: Use Google AI Platform’s AutoML capabilities to automate model training and hyperparameter tuning, saving time and resources.
- Implement Continuous Integration/Continuous Deployment (CI/CD): Integrate CI/CD pipelines with GKE to streamline the deployment of ML models and ensure rapid iteration.
- Monitor and Manage Models: Employ monitoring tools like TensorBoard and Stackdriver to track model performance and diagnose real-time issues.
- Ensure Security and Compliance: Utilize GCP’s security features to protect data and models and adhere to relevant compliance standards.
5. Real-world examples of Scalable ML Models on GCP
Several organizations have successfully leveraged GCP to build and deploy scalable ML models:
- Spotify: Uses GCP to analyze user data and personalize music recommendations, scaling their models to handle millions of users.
- Airbnb: Deploys ML models on GCP to optimize pricing strategies, dynamically adjusting based on market trends and demand.
- Snap Inc.: Utilizes GCP’s AI and ML services to enhance image recognition and improve user engagement through tailored content.
These examples demonstrate the versatility and scalability of GCP in handling diverse ML workloads across various industries.
6. Recommended Books and Courses
Enhance your expertise in building scalable machine-learning models with GCP by exploring these recommended resources:
Books:
- *"Google Cloud Platform for Architects"* by Vitthal Srinivasan
A comprehensive guide to designing and implementing solutions on GCP, including ML applications. [Buy on Amazon]
- *"Architecting Modern Data Platforms"* by Jan Kunigk, Ian Buss, Paul Wilkinson, and Lars George
Learn how to build scalable data platforms on GCP with practical examples. [Buy on Amazon]
Courses:
- *GCP Machine Learning Engineer Certification Courses*
Prepare for the Machine Learning Engineer certification with in-depth courses covering GCP’s ML services. [Enroll in GCP ML Engineer Courses](https://www.coursera.org/google-cloud)
GCP Services:
- *Google AI Platform*
Streamline your ML workflows with GCP’s integrated AI tools. [Subscribe to Google AI Platform](https://cloud.google.com/ai-platform)
- *BigQuery*
Efficiently manage and analyze large datasets with BigQuery. [Get BigQuery](https://cloud.google.com/bigquery)
7. Conclusion
Building scalable machine learning models is pivotal for organizations seeking to harness the full potential of their data. Google Cloud Platform provides a robust and flexible environment with advanced tools and services tailored for scalable ML development. By leveraging GCP’s AI Platform, BigQuery, and Kubernetes Engine, data scientists and engineers can efficiently build, deploy, and manage ML models that grow with their data and business needs.
Adhering to best practices, such as optimizing data storage, implementing CI/CD pipelines, and ensuring robust security, further enhances the scalability and reliability of your ML models on GCP. As real-world examples demonstrate, GCP empowers organizations across various industries to achieve their ML objectives effectively.
Investing in recommended books and courses can accelerate your learning and proficiency in using GCP for scalable machine learning. Embrace the power of Google Cloud Platform to elevate your machine learning projects and drive meaningful insights for your organization.
Start building scalable machine learning models today with Google Cloud Platform and unlock the potential to transform your data into actionable intelligence.
8. Disclaimer
*Disclaimer: This post contains affiliate links, meaning we may earn a commission if you click a link and make a purchase at no additional cost. We only recommend products and services we believe will add value to our readers.*
Comments
Please log in to leave a comment.
No comments yet.