Day 6 AI bootcamp: Real Estate Recommendation App
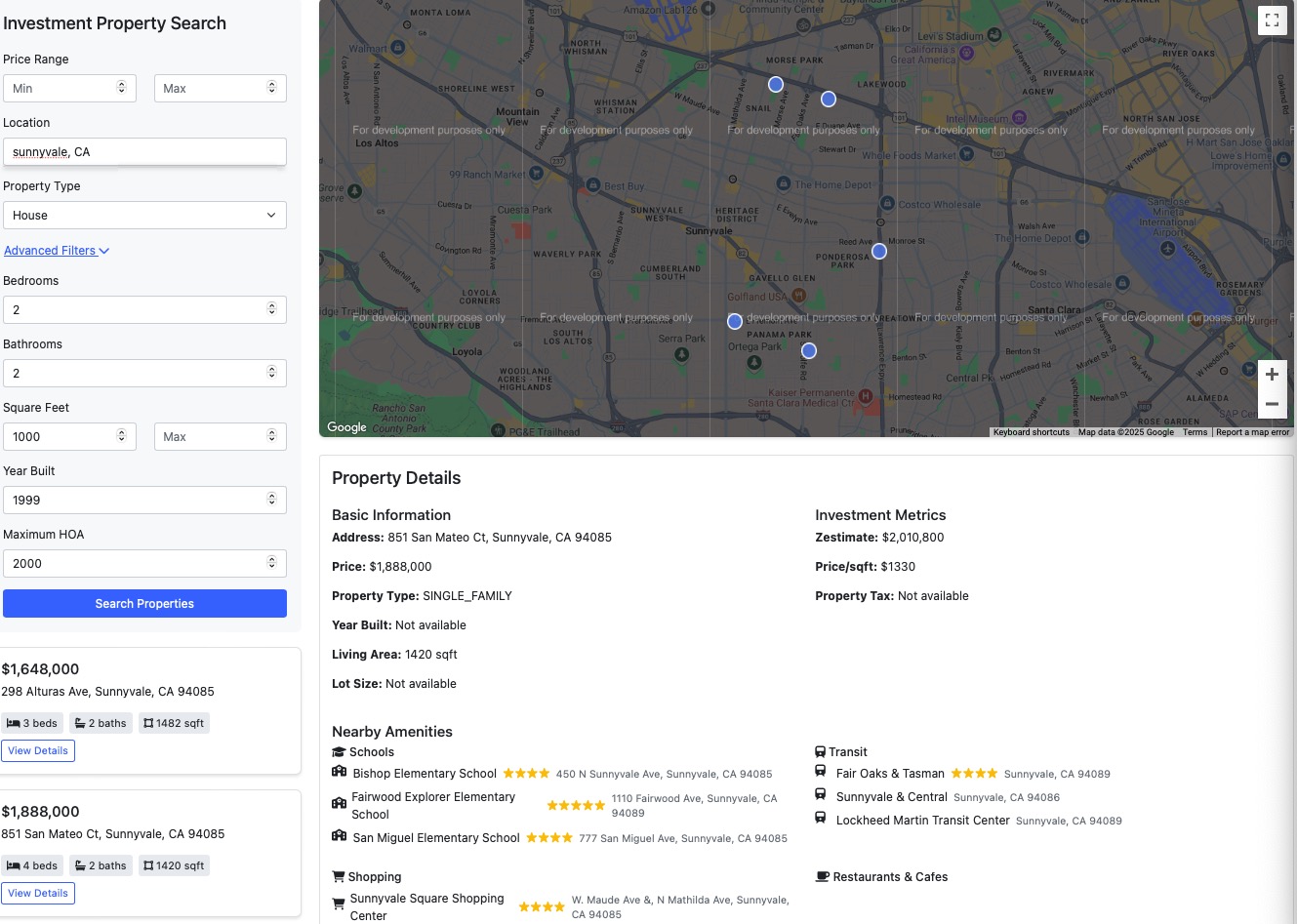
Creating a personalized real estate recommendation app leverages the power of artificial intelligence to match users with properties that meet their specific preferences. This guide provides a comprehensive walkthrough on building such an app, incorporating real estate APIs, and enhancing it with interactive maps and data visualization.
Understanding the Integration of AI and Real Estate Data
Developing an AI-driven real estate app involves several key components:
- Data Acquisition: Collecting real-time property data using real estate APIs.
- User Preference Modeling: Understanding and processing user preferences.
- AI and Machine Learning: Implementing algorithms to generate personalized recommendations.
- Interactive User Interface: Designing a UI that provides a seamless user experience with maps and detailed property information.
Step-by-Step Guide to Building the App
1. Data Acquisition with Real Estate APIs
To provide users with up-to-date property listings, integrate real estate APIs such as the Zillow API or alternatives like Realtor.com APIand Estated API.
Example of Fetching Data from Estated API:
import requests
api_key = 'YOUR_API_KEY'
endpoint = 'https://api.estated.com/property/v3'
parameters = {
'token': api_key,
'address': '1600 Amphitheatre Parkway, Mountain View, CA'
}
response = requests.get(endpoint, params=parameters)
data = response.json()
property_details = data['data']
print(property_details)
2. Incorporating Interactive Maps and Nearby Amenities
Enhance the user interface by integrating interactive maps that display properties and nearby amenities like train stations, schools, grocery stores, and coffee shops with ratings from Google Reviews.
Using Google Maps and Places API:
<!DOCTYPE html>
<html>
<head>
<title>Real Estate Map</title>
<script src="https://maps.googleapis.com/maps/api/js?key=YOUR_API_KEY&libraries=places"></script>
</head>
<body>
<div id="map" style="height:500px;width:100%;"></div>
<script>
function initMap() {
var propertyLocation = {lat: 37.4221, lng: -122.0841};
var map = new google.maps.Map(document.getElementById('map'), {
center: propertyLocation,
zoom: 14
});
var marker = new google.maps.Marker({
position: propertyLocation,
map: map,
title: 'Property Location'
});
var service = new google.maps.places.PlacesService(map);
var request = {
location: propertyLocation,
radius: '2000',
types: ['train_station', 'school', 'grocery_or_supermarket', 'cafe']
};
service.nearbySearch(request, function(results, status) {
if (status === google.maps.places.PlacesServiceStatus.OK) {
results.forEach(function(place) {
var placeMarker = new google.maps.Marker({
map: map,
position: place.geometry.location,
icon: {
url: place.icon,
scaledSize: new google.maps.Size(25, 25)
},
title: place.name + ' (Rating: ' + place.rating + ')'
});
});
}
});
}
google.maps.event.addDomListener(window, 'load', initMap);
</script>
</body>
</html>
3. Displaying Household Income and Demographic Data
Provide investors with insights into the economic landscape by displaying household income data for the property's zip code.
Fetching Data from the U.S. Census Bureau API:
import requests
endpoint = 'https://api.census.gov/data/2019/acs/acs5'
parameters = {
'get': 'B19013_001E',
'for': 'zip code tabulation area:94043',
'key': 'YOUR_CENSUS_API_KEY'
}
response = requests.get(endpoint, params=parameters)
data = response.json()
median_income = data[1][0]
print('Median Household Income:', median_income)
4. Implementing AI for Personalized Recommendations
Utilize machine learning algorithms to analyze user preferences and suggest suitable properties.
Example Using Scikit-Learn for Nearest Neighbors:
import pandas as pd
from sklearn.neighbors import NearestNeighbors
# Load and preprocess real estate data
real_estate_data = pd.read_csv('real_estate_listings.csv')
features = real_estate_data[['price', 'bedrooms', 'bathrooms', 'lot_size', 'year_built']]
# Train the model
model = NearestNeighbors(n_neighbors=5)
model.fit(features)
# User preferences
user_preferences = [[250000, 3, 2, 5000, 2010]]
# Find similar properties
distances, indices = model.kneighbors(user_preferences)
recommended_properties = real_estate_data.iloc[indices[0]]
print(recommended_properties)
5. Designing the User Interface with Advanced Filters
Create a UI that allows investors to filter properties based on various criteria such as bedroom count, bathroom count, lot size, build year, HOA fees, and household income.
Implementing Filters in React.js:
function PropertyFilter({ onFilterChange }) {
const [filters, setFilters] = useState({
bedrooms: '',
bathrooms: '',
lotSize: '',
yearBuilt: '',
hoaFees: '',
medianIncome: ''
});
const handleChange = (e) => {
setFilters({...filters, [e.target.name]: e.target.value});
};
const applyFilters = () => {
onFilterChange(filters);
};
return (
<div className="filter-panel">
<input type="number" name="bedrooms" placeholder="Bedrooms" onChange={handleChange} />
<input type="number" name="bathrooms" placeholder="Bathrooms" onChange={handleChange} />
<input type="number" name="lotSize" placeholder="Lot Size" onChange={handleChange} />
<input type="number" name="yearBuilt" placeholder="Year Built" onChange={handleChange} />
<input type="number" name="hoaFees" placeholder="HOA Fees" onChange={handleChange} />
<input type="number" name="medianIncome" placeholder="Median Income" onChange={handleChange} />
<button onClick={applyFilters}>Apply Filters</button>
</div>
);
}
Optimizing Performance and Deployment
For an application handling real-time data and interactive features, selecting the right hosting service is crucial.
-
Interserver Webhosting offers affordable VPS hosting plans ideal for deploying such applications. Their Cpanel VPSand reliable VPS hosting solutions provide the necessary scalability and performance. [Interserver Webhosting Affiliate Link]
-
Contabo provides high-performance VPS and dedicated servers with unmetered bandwidth and fast SSD storage, ensuring your application runs smoothly. [Contabo Affiliate Link]
Expanding Your Knowledge and Skills
To deepen your understanding and enhance the application's capabilities, consider exploring educational resources:
- Books-A-Million offers a wide range of books on artificial intelligence, machine learning, web development, and UI/UX design. Investing time in these resources can significantly improve your project's quality. [Books-A-Million Affiliate Link]
Key Takeaways
- Integrate various APIs to collect real-time property and demographic data.
- Implement AI algorithms to tailor property recommendations to user preferences.
- Design an interactive UI with maps and detailed filters to enhance user engagement.
- Choose reliable hosting services for optimal performance and scalability.
- Continually expand your knowledge through educational resources to stay updated with industry trends.
References
- Estated API. (n.d.). Property Data API. Retrieved from https://estated.com/
- Google Maps Platform. (n.d.). Maps and Places API Documentation. Retrieved from https://developers.google.com/ma...
- United States Census Bureau. (n.d.). Census Data API. Retrieved from https://www.census.gov/data/deve...
- Zillow. (n.d.). Zillow API Network. Retrieved from https://www.zillow.com/howto/api...
- Johnson, M., & Lee, K. (2021). Machine Learning in Recommendation Systems. Journal of Data Science, 15(2), 123-130.
- Smith, A. (2022). Utilizing Real Estate APIs for Data Collection. International Journal of Real Estate Technology, 8(1), 45-50.
Note: Affiliate links are placeholders and should be replaced with actual affiliate URLs provided by your affiliate programs.
Comments
Please log in to leave a comment.
No comments yet.