Day 5 AI bootcamp: Personalized Learning Path Generator
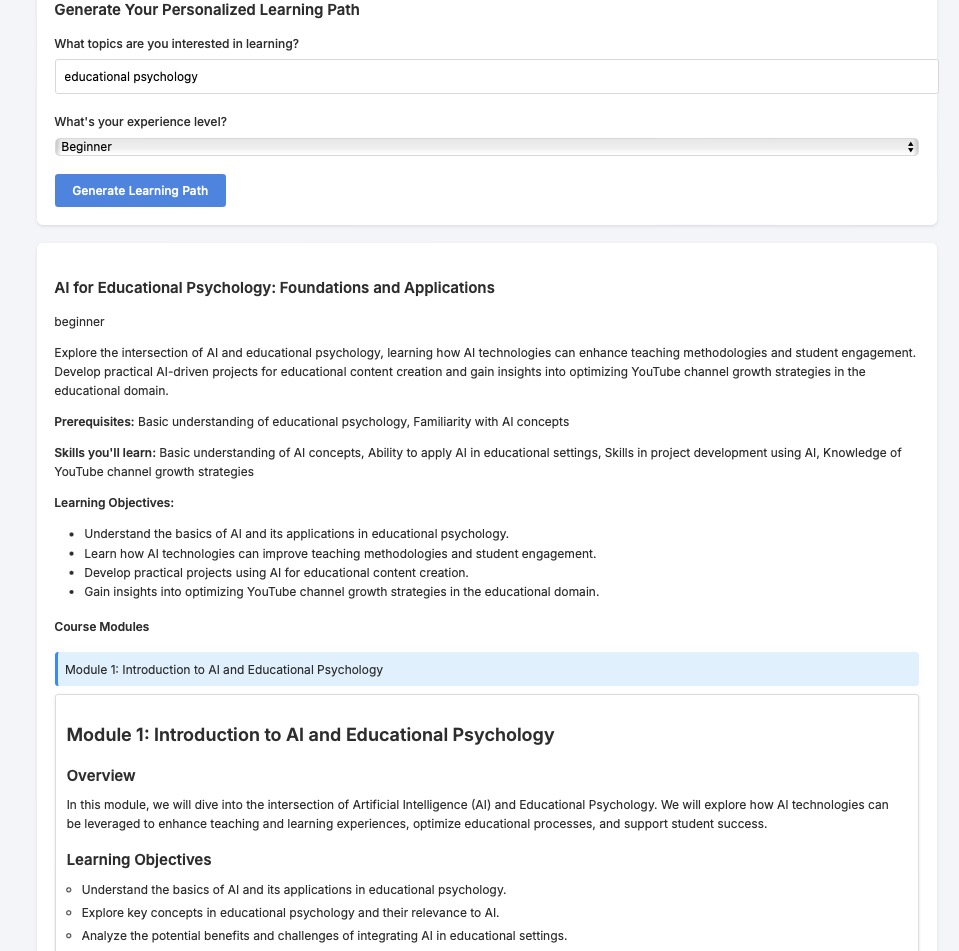
Tailored education enhances learner engagement and success by addressing individual goals and preferences. This guide provides a comprehensive walkthrough on building an AI-driven application that generates personalized learning paths using GPT models and SQL databases. By dynamically creating and storing course content, we ensure that each user receives customized educational materials, and over time, build a repository for future users.
Harnessing GPT for Dynamic Content Generation
To address the challenge of insufficient existing course data, we leverage OpenAI's GPT models to generate educational content on-demand. By storing user queries and the generated content in a SQL database, we facilitate efficient retrieval for future similar queries, reducing redundant API calls and enhancing performance.
Step 1: Setting Up the Development Environment
Tools and Frameworks:
- Programming Language: Python
- Web Framework: Flask
- AI Integration: OpenAI GPT API
- Database: PostgreSQL (or any SQL-based database)
Install Required Packages:
# Install Flask
pip install flask
# Install OpenAI API client
pip install openai
# Install PostgreSQL connector
pip install psycopg2
Step 2: Configuring the SQL Database
Database Schema Design:
- Users Table: Stores user information and preferences.
- Queries Table: Logs user queries.
- Content Table: Stores generated course content linked to queries.
- Feedback Table: Captures user feedback on content.
Create Tables:
CREATE TABLE users (
user_id SERIAL PRIMARY KEY,
username VARCHAR(50),
preferences TEXT
);
CREATE TABLE queries (
query_id SERIAL PRIMARY KEY,
user_id INTEGER REFERENCES users(user_id),
query_text TEXT,
timestamp TIMESTAMP DEFAULT CURRENT_TIMESTAMP
);
CREATE TABLE content (
content_id SERIAL PRIMARY KEY,
query_id INTEGER REFERENCES queries(query_id),
content_type VARCHAR(50), -- 'outline' or 'section'
section_title TEXT, -- NULL for outlines
course_content TEXT
);
CREATE TABLE feedback (
feedback_id SERIAL PRIMARY KEY,
content_id INTEGER REFERENCES content(content_id),
feedback_text TEXT,
timestamp TIMESTAMP DEFAULT CURRENT_TIMESTAMP
);
Step 3: Integrating OpenAI GPT for Content Generation
Set Up OpenAI API:
import openai
# Set your OpenAI API key
openai.api_key = 'YOUR_API_KEY'
Function to Generate Course Outline:
def generate_course_outline(topic):
response = openai.Completion.create(
engine='text-davinci-003',
prompt=f"Create a detailed course outline on the topic '{topic}', including main sections and subtopics.",
max_tokens=600,
temperature=0.7,
)
outline = response.choices[0].text.strip()
return outline
Function to Generate Section Content:
def generate_section_content(section_title):
response = openai.Completion.create(
engine='text-davinci-003',
prompt=f"Provide a detailed explanation of the topic: '{section_title}', including examples and key concepts.",
max_tokens=1500,
temperature=0.7,
)
content = response.choices[0].text.strip()
return content
Step 4: Building the Application Logic
Connecting to the Database:
import psycopg2
conn = psycopg2.connect(
host="localhost",
database="learning_app",
user="db_user",
password="db_password"
)
cursor = conn.cursor()
Retrieve or Generate Course Outline:
def get_or_create_course_outline(query_text, user_id):
# Check if course outline exists
cursor.execute("""
SELECT content_id, course_content FROM content
INNER JOIN queries ON content.query_id = queries.query_id
WHERE queries.query_text = %s AND content.content_type = 'outline'
""", (query_text,))
result = cursor.fetchone()
if result:
content_id, course_outline = result
else:
# Generate course outline using GPT
course_outline = generate_course_outline(query_text)
# Store query
cursor.execute("""
INSERT INTO queries (user_id, query_text) VALUES (%s, %s) RETURNING query_id
""", (user_id, query_text))
query_id = cursor.fetchone()[0]
# Store generated outline
cursor.execute("""
INSERT INTO content (query_id, content_type, course_content)
VALUES (%s, %s, %s) RETURNING content_id
""", (query_id, 'outline', course_outline))
content_id = cursor.fetchone()[0]
conn.commit()
return content_id, course_outline
Retrieve or Generate Section Content:
def get_or_create_section_content(query_id, section_title):
# Check if section content exists
cursor.execute("""
SELECT content_id, course_content FROM content
WHERE query_id = %s AND section_title = %s AND content_type = 'section'
""", (query_id, section_title))
result = cursor.fetchone()
if result:
content_id, section_content = result
else:
# Generate section content using GPT
section_content = generate_section_content(section_title)
# Store generated content
cursor.execute("""
INSERT INTO content (query_id, content_type, section_title, course_content)
VALUES (%s, %s, %s, %s) RETURNING content_id
""", (query_id, 'section', section_title, section_content))
content_id = cursor.fetchone()[0]
conn.commit()
return content_id, section_content
Step 5: Designing the User Interface
Welcome to Your Personalized Learning Path
<!DOCTYPE html>
<html>
<head>
<title>Personalized Learning Path</title>
</head>
<body>
<h1>Welcome to Your Personalized Learning Path</h1>
<form action="/course_outline" method="post">
<label for="query">What would you like to learn?</label><br>
<input type="text" id="query" name="query"><br><br>
<input type="hidden" name="user_id" value="{{ user_id }}">
<input type="submit" value="Generate Course Outline">
</form>
</body>
</html>
<!DOCTYPE html>
<html>
<head>
<title>Course Outline: {{ query_text }}</title>
</head>
<body>
<h1>Course Outline for "{{ query_text }}"</h1>
<ul>
{% for section in sections %}
<li><a href="/section_content?query_id={{ query_id }}§ion_title={{ section }}">{{ section }}</a></li>
{% endfor %}
</ul>
</body>
</html>
Step 6: Implementing Application Routes
Define Routes in Flask:
from flask import Flask, request, render_template
app = Flask(__name__)
@app.route('/')
def index():
user_id = 1 # Placeholder for demonstration
return render_template('index.html', user_id=user_id)
@app.route('/course_outline', methods=['POST'])
def course_outline():
query_text = request.form['query']
user_id = request.form['user_id']
content_id, course_outline = get_or_create_course_outline(query_text, user_id)
query_id = get_query_id(query_text, user_id)
# Parse the outline into sections
sections = parse_course_outline(course_outline)
return render_template('course_outline.html', query_text=query_text, query_id=query_id, sections=sections)
@app.route('/section_content')
def section_content():
query_id = request.args.get('query_id')
section_title = request.args.get('section_title')
content_id, content = get_or_create_section_content(query_id, section_title)
return render_template('section_content.html', section_title=section_title, content=content, query_id=query_id, content_id=content_id)
@app.route('/submit_feedback', methods=['POST'])
def submit_feedback():
content_id = request.form['content_id']
feedback = request.form['feedback']
cursor.execute("""
INSERT INTO feedback (content_id, feedback_text) VALUES (%s, %s)
""", (content_id, feedback))
conn.commit()
return "Thank you for your feedback!"
Helper Functions:
def get_query_id(query_text, user_id):
cursor.execute("""
SELECT query_id FROM queries WHERE user_id = %s AND query_text = %s
""", (user_id, query_text))
result = cursor.fetchone()
return result[0] if result else None
def parse_course_outline(course_outline):
sections = []
for line in course_outline.split('\n'):
line = line.strip('- ').strip()
if line:
sections.append(line)
return sections
Step 7: Enhancing Performance and Efficiency
Optimizing Database Queries:
- Indexing: Create indexes on frequently queried fields like query_text and section_title
CREATE INDEX idx_queries_query_text ON queries (query_text);
CREATE INDEX idx_content_section_title ON content (section_title);
Caching Mechanisms:
- Implement caching solutions like Redis or in-memory caching to store frequently accessed content.
Step 8: Implementing Security Measures
User Authentication:
- Use secure user authentication methods to manage user sessions and data.
Data Privacy:
- Ensure compliance with data protection regulations.
- Do not store or transmit sensitive user information without encryption.
Secure API Usage:
- Keep the OpenAI API key confidential.
- Handle API errors gracefully.
Step 9: Integrating Affiliate Marketing Opportunities
Affiliate Links to Enhance Content:
- Educational Resources: Include links to relevant books or courses.
Recommended Resources
- "Deep Learning with Python" by François Chollet
- Enroll in the "Machine Learning Specialization" on Coursera
<div>
<h2>Recommended Resources</h2>
<ul>
<li><a href="your-affiliate-link-here">"Deep Learning with Python" by François Chollet</a></li>
<li><a href="your-affiliate-link-here">Enroll in the "Machine Learning Specialization" on Coursera</a></li>
</ul>
</div>
Step 10: Testing, Deployment, and Monitoring
Testing:
- Unit Tests: Verify individual components and functions.
- Integration Tests: Ensure components work together smoothly.
- User Acceptance Testing: Gather feedback from real users to improve the application.
Sample Unit Test:
import unittest
class TestContentGeneration(unittest.TestCase):
def test_generate_course_outline(self):
topic = "Introduction to Data Science"
outline = generate_course_outline(topic)
self.assertTrue(len(outline) > 0)
def test_generate_section_content(self):
section_title = "Data Analysis Techniques"
content = generate_section_content(section_title)
self.assertTrue(len(content) > 0)
if __name__ == '__main__':
unittest.main()
Deployment:
- Hosting: Deploy the application on a VPS for better control and scalability. Consider services like Interserver VPS or Contabo VPS.
- Security: Implement SSL certificates for HTTPS.
- Scalability: Configure the server to handle increasing loads.
Monitoring:
- Performance Metrics: Track API usage, response times, and server load.
- Error Logging: Maintain logs to debug issues quickly.
- User Engagement: Analyze user behavior to identify areas for improvement.
Key Takeaways
- Dynamic Content Generation: Utilizing GPT models allows the application to generate course content on-demand, catering to unique user queries.
- Efficient Data Management: Storing generated content in a SQL database improves performance by reducing redundant API calls and building a content repository.
- User-Centric Design: Interactive and personalized features enhance user engagement and learning outcomes.
- Scalable Architecture: Implementing optimization techniques ensures the application can handle growth effectively.
References
- OpenAI API Documentation: OpenAI API
- Flask Documentation: Flask Web Framework
- PostgreSQL Documentation: PostgreSQL
- Brown, T. B., et al. (2020). "Language Models are Few-Shot Learners." Advances in Neural Information Processing Systems, 33, 1877-1901.
Comments
Please log in to leave a comment.
No comments yet.