Day 3 AI bootcamp: Fashion Stylist App with Flask
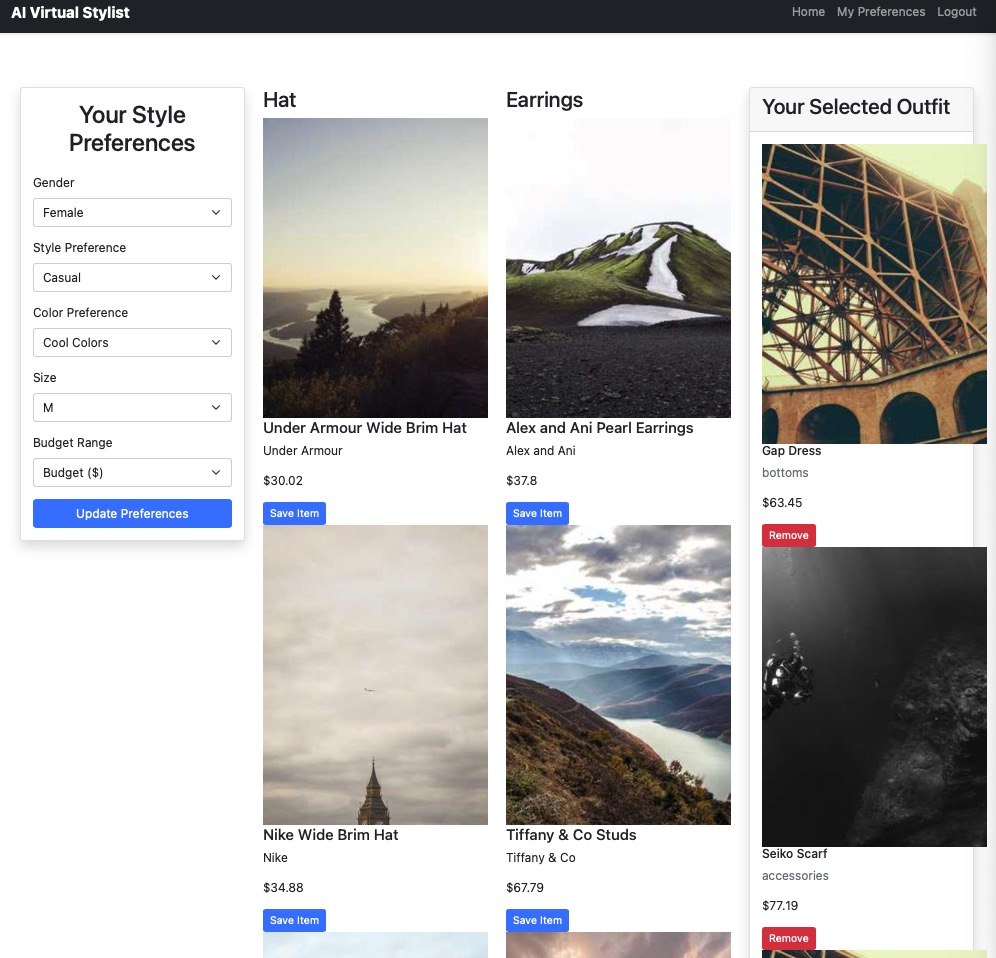
This guide provides detailed instructions, code examples, and diagrams to help you build an AI-powered virtual stylist app that offers personalized fashion recommendations. By integrating AI tools and the Amazon Fashion Product API, you can create an application that not only suggests outfits based on user preferences but also allows users to purchase items directly, incorporating affiliate marketing opportunities.
Integrating AI and Fashion: A Technical Approach
Developing a virtual stylist app involves combining machine learning algorithms with real-time product data to provide personalized clothing recommendations. By leveraging your expertise in data engineering and machine learning—gained from working with companies like Yahoo and MadHive—you can build a scalable and efficient application.
Setting Up the Development Environment
- Programming Language: Python 3.x
- Web Framework: Flask or Django
- Machine Learning Libraries: TensorFlow, PyTorch
- Data Platforms: AWS, Google Cloud Platform (BigQuery), or Azure
- Database: PostgreSQL or MongoDB
- APIs: Amazon Product Advertising API
- Frontend Technologies: HTML, CSS, JavaScript
Prerequisites
- Amazon Product Advertising API Access: Sign up for access and obtain API credentials.
- Amazon Associates Program: Register to get affiliate tags for generating commission on product sales.
- AWS SDK for Python (Boto3): Install for interacting with the Amazon API.
Workflow Overview
1. User Interaction: Collect user preferences via a web interface.
2. Data Processing: Use machine learning to analyze preferences.
3. API Integration: Fetch product data from Amazon based on analysis.
4. Recommendation Generation: Present personalized outfit suggestions.
5. Affiliate Integration: Provide purchase links with affiliate tracking.
Developing the Frontend Interface
Create a user-friendly interface to collect preferences such as style, size, color, and occasion.
```html
<form action="/recommend" method="post">
<label for="style">Preferred Style:</label>
<select name="style" id="style">
<option value="casual">Casual</option>
<option value="formal">Formal</option>
<!-- Additional options -->
</select>
<!-- Additional input fields -->
<button type="submit">Get Recommendations</button>
</form>
```
Building the Backend with Flask
Set up routes to handle user input and generate recommendations.
Flask App Initialization
```python
from flask import Flask, request, render_template
app = Flask(__name__)
@app.route('/')
def index():
return render_template('index.html')
@app.route('/recommend', methods=['POST'])
def recommend():
preferences = {
'style': request.form.get('style'),
'color': request.form.get('color'),
# Additional preferences
}
recommendations = generate_recommendations(preferences)
return render_template('recommendations.html', items=recommendations)
if __name__ == '__main__':
app.run(debug=True)
```
#Developing the Machine Learning Model
#Utilize your experience with supervised and unsupervised learning to build a model that predicts suitable clothing items.
#Model Training with TensorFlow
```python
import tensorflow as tf
from tensorflow.keras.models import Sequential
from tensorflow.keras.layers import Dense
def create_model(input_dim):
model = Sequential()
model.add(Dense(128, activation='relu', input_dim=input_dim))
model.add(Dense(64, activation='relu'))
model.add(Dense(number_of_categories, activation='softmax'))
model.compile(optimizer='adam', loss='categorical_crossentropy', metrics=['accuracy'])
return model
# Assume X_train and y_train are preprocessed datasets
model = create_model(X_train.shape[1])
model.fit(X_train, y_train, epochs=20, batch_size=32)
```
#Integrating the Amazon Fashion Product API
#Fetch real-time product data based on model predictions.
#Fetching Products from Amazon API
```python
import requests
import hashlib
import hmac
import time
def generate_amazon_url(keywords):
# Code to generate signed URL for Amazon API request
# Include your Access Key, Secret Key, and Affiliate Tag
pass
def fetch_products(keywords):
url = generate_amazon_url(keywords)
response = requests.get(url)
if response.status_code == 200:
products = parse_response(response.content)
return products
else:
return []
def parse_response(response_content):
# Parse XML or JSON response from Amazon
# Extract necessary product information
pass
```
#Generating Recommendations
#Combine user preferences, model predictions, and API data.
#Recommendation Function
```python
def generate_recommendations(preferences):
# Predict categories using the ML model
predicted_categories = model.predict([process_preferences(preferences)])
# Convert predictions to keywords
keywords = categories_to_keywords(predicted_categories)
# Fetch products from Amazon API
products = fetch_products(keywords)
# Generate affiliate links
for product in products:
product['affiliate_link'] = get_affiliate_link(product['url'])
return products
```
Optimizing Data Processing and Machine Learning
Leverage your proficiency with big data tools like PySpark and expertise in cloud platforms to handle large datasets and improve model performance.
Data Pipeline Optimization
- Use PySpark for efficient data processing.
- Store datasets in cloud storage (e.g., AWS S3 or Google Cloud Storage).
- Utilize distributed computing for model training.
Ensuring Data Security and Compliance
Implement secure authentication and data handling practices.
- Use HTTPS for all data transmissions.
- Employ OAuth 2.0 for user authentication if necessary.
- Follow GDPR and other data protection regulations.
Testing and Deployment
- Automated Testing: Use unit tests to ensure code reliability.
- Continuous Integration: Implement CI/CD pipelines for seamless deployment.
- Scalability: Deploy using services like AWS Elastic Beanstalk or Google App Engine.
- Hosting Services: Recommend reliable hosting providers like [InterServer] and [Contabo] for deploying applications.
- Educational Resources: Suggest books such as "Hands-On Machine Learning with Scikit-Learn, Keras, and TensorFlow" available on Amazon.
Insights from Building the App
Developing this AI-powered virtual stylist app demonstrates how machine learning and API integration can create personalized user experiences. Incorporating affiliate marketing not only enhances functionality but also provides a revenue stream.
References
- Amazon Web Services, Inc. (n.d.). *Amazon Product Advertising API 5.0 Reference Guide*. Retrieved from https://webservices.amazon.com/paapi5/documentation/
- Géron, A. (2019). *Hands-On Machine Learning with Scikit-Learn, Keras, and TensorFlow* (2nd ed.). O'Reilly Media.
Final Thoughts on Creating an AI-Powered Virtual Stylist
By integrating advanced AI algorithms with real-time data from the Amazon Fashion API, you can deliver personalized fashion recommendations that enhance user engagement. Leveraging affiliate marketing amplifies the app's commercial potential, transforming it into a viable business opportunity.
---
This guide combines technical expertise in machine learning, data processing, and web development to help you build a sophisticated virtual stylist app. By following these steps and utilizing the provided code examples, you can create an application that offers value to users and opens up avenues for monetization through affiliate marketing.
Comments
Please log in to leave a comment.
No comments yet.