Day 1 AI bootcamp: Movie Recommendation Web App
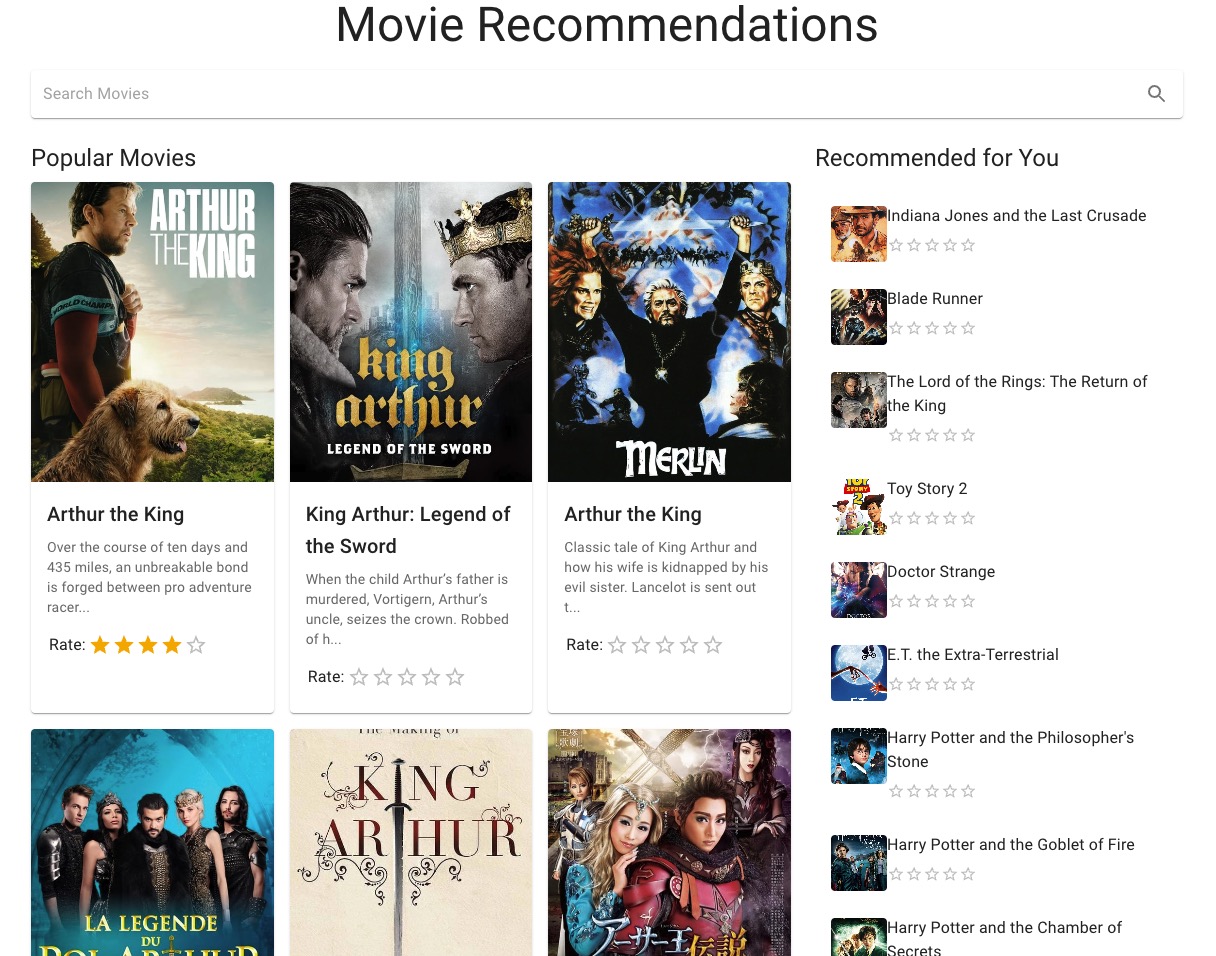
Creating a personalized movie recommendation application involves leveraging movie APIs and artificial intelligence to deliver tailored suggestions to users. This guide walks through building the front end, integrating AI for recommendations, incorporating user feedback for dynamic updates, and enhancing recommendation algorithms with machine learning models. Alongside, you'll find code examples, diagrams, and resources to help you develop a comprehensive understanding of the process.
### Exploring the Development of a Personalized Movie Recommendation System
Developing an AI-driven movie recommendation app starts with understanding the foundational technologies and methodologies. Utilizing APIs like The Movie Database (TMDb) and integrating AI models can significantly enhance user experience by providing customized movie suggestions based on individual preferences.
### Tracing the Evolution of Recommendation Systems in Modern Applications
Recommendation systems have evolved from simple collaborative filtering techniques to complex AI and machine learning algorithms. Early systems relied heavily on user ratings and item popularity, but today's applications use sophisticated models considering various factors, including user behavior, contextual information, and real-time feedback.
### Understanding the Core Components of an AI-Based Recommendation Engine
At the heart of a movie recommendation app are several key components:
- Front-End Interface: The user interface where users interact with the app, browse movies, and receive recommendations.
- Movie APIs: Services like TMDb that provide access to vast databases of movie information.
- AI Integration: Leveraging machine learning algorithms to analyze user data and generate personalized recommendations.
- User Feedback Mechanism: Allowing users to rate movies, which the system uses to refine future suggestions.
### Implementing Personalized Recommendations with Practical Code Examples
To build the front-end and integrate AI for recommendations, follow these steps:
1. Set Up the Front-End Framework: Use React.js for a dynamic user interface.
2. Connect to a Movie API: Fetch movie data using TMDb API.
3. Integrate AI for Recommendations: Implement a machine learning model to analyze user preferences and suggest movies.
Here's a sample React component that fetches and displays movie data:
```javascript
import React, { useState, useEffect } from 'react';
import axios from 'axios';
const MovieList = () => {
const [movies, setMovies] = useState([]);
useEffect(() => {
axios.get('https://api.themoviedb.org/3/movie/popular', {
params: { api_key: 'YOUR_TMDB_API_KEY' }
})
.then(response => {
setMovies(response.data.results);
})
.catch(error => {
console.error('Error fetching movies:', error);
});
}, []);
return (
<div>
<h1>Popular Movies</h1>
<ul>
{movies.map(movie => (
<li key={movie.id}>
{movie.title} - Rating: {movie.vote_average}
</li>
))}
</ul>
</div>
);
};
export default MovieList;
```
### Enhancing Recommendations Through User Interaction
Incorporating user feedback is crucial for refining recommendations. Allow users to rate movies and use these ratings to adjust future suggestions dynamically.
Implementing User Rating Feature:
```javascript
const handleRating = (movieId, rating) => {
axios.post('/api/rate', { movieId, rating })
.then(response => {
// Update recommendations based on new rating
})
.catch(error => {
console.error('Error submitting rating:', error);
});
};
```
Flowchart of User Feedback Integration:
```mermaid
flowchart TD
A[User Rates a Movie] --> B[Send Rating to Server]
B --> C[Update User Profile]
C --> D[Recompute Recommendations]
D --> E[Display Updated Recommendations]
```
### Leveraging Machine Learning Models for Advanced Recommendations
To further enhance the recommendation system, integrate machine learning models such as collaborative or content-based filtering. These models analyze user interactions and movie attributes to predict and suggest movies that align with user preferences.
Example: Collaborative Filtering with Python's Surprise Library
```python
from surprise import Dataset, Reader, SVD
from surprise.model_selection import train_test_split
# Load dataset
reader = Reader(rating_scale=(1, 5))
data = Dataset.load_from_df(ratings_df[['userId', 'movieId', 'rating']], reader)
# Split data
trainset, testset = train_test_split(data, test_size=0.2)
# Train model
model = SVD()
model.fit(trainset)
# Predict
predictions = model.test(testset)
```
### Key Insights for Developing an Effective Recommendation System
Building an effective AI-powered movie recommendation app requires seamless integration of front-end development, API utilization, machine learning, and user engagement strategies. The system can provide increasingly accurate and personalized movie suggestions by continuously incorporating user feedback and refining machine-learning models.
### Further Reading and Resources
For a deeper dive into building recommendation systems and enhancing your app with AI, consider the following resources:
- "Hands-On Machine Learning with Scikit-Learn, Keras, and TensorFlow" by Aurélien Géron
[Purchase on Amazon]
- "Building Machine Learning Powered Applications" by Emmanuel Ameisen
[Purchase on Amazon]
- "React – Up & Running" by Stoyan Stefanov
[Purchase on Amazon]
The Movie Database. (n.d.). *TMDb API Documentation*. Retrieved from [https://developers.themoviedb.org/3/getting-started/introduction]
Comments
Please log in to leave a comment.
No comments yet.