A Beginner’s Guide to Machine Learning with Python
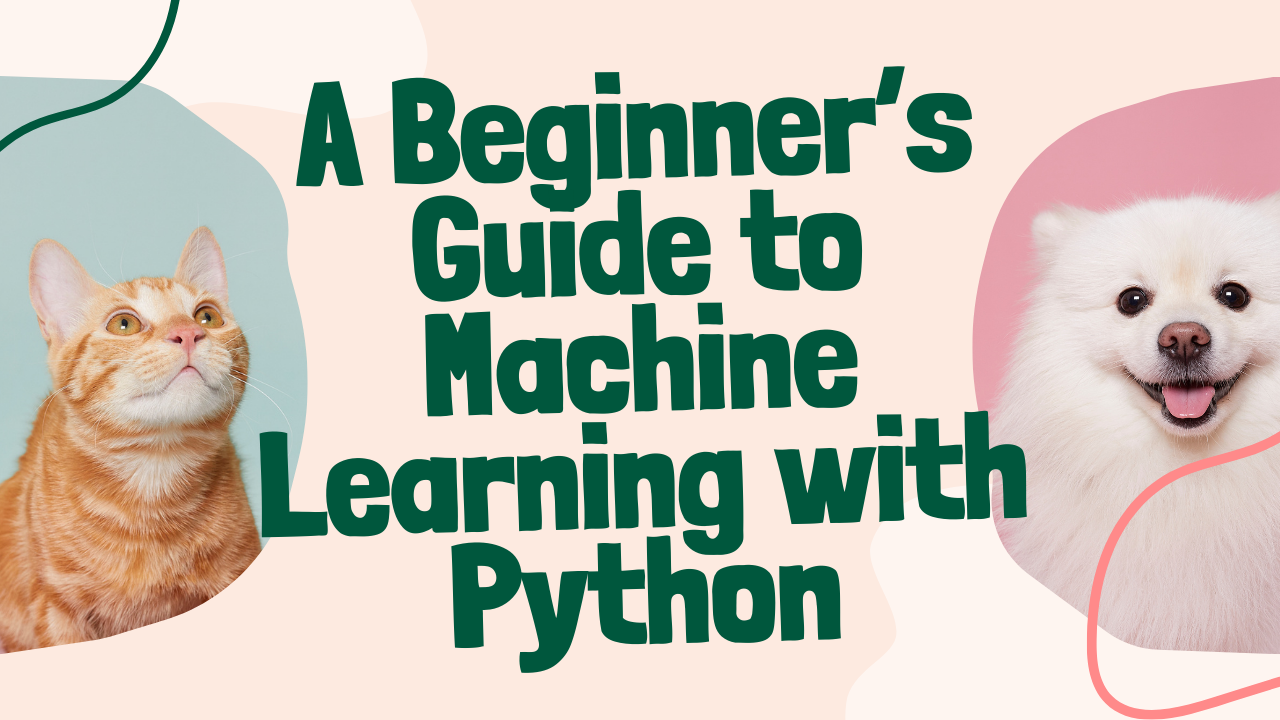
Welcome to the exciting world of machine learning! Whether you're a complete novice or an aspiring data scientist, this guide is designed to help you embark on your machine learning journey using Python. Machine learning is transforming industries by enabling computers to learn from data and make informed decisions. With Python’s simplicity and powerful libraries, getting started has never been easier. In this comprehensive guide, we will cover fundamental concepts, essential libraries, and simple projects to kickstart your machine learning adventure. Additionally, we’ll introduce some valuable resources and tools that can accelerate your learning process.
1. Introduction to Machine Learning
Machine learning (ML) is a subset of artificial intelligence (AI) that focuses on developing algorithms that allow computers to learn from and make predictions or decisions based on data. Unlike traditional programming, where rules are explicitly coded, machine learning algorithms identify patterns and relationships within data to improve their performance over time.
Key areas within machine learning include:
- Supervised Learning: The algorithm learns from labeled data.
- Unsupervised Learning: The algorithm identifies patterns in unlabeled data.
- Reinforcement Learning: The algorithm learns by interacting with an environment to achieve a goal.
Understanding these concepts is essential as you delve deeper into machine learning.
---
2. Why Python for Machine Learning?
Python has become the go-to language for machine learning due to its simplicity, readability, and vast ecosystem of libraries and frameworks. Its versatility allows beginners to prototype solutions and experts to build complex models efficiently and quickly. Here are some reasons why Python is ideal for machine learning:
- Ease of Learning: Python’s syntax is straightforward, making it accessible for beginners.
- Extensive Libraries: Python boasts a rich collection of libraries optimized for machine learning tasks.
- Community Support: A large, active community ensures continuous improvements and abundant learning resources.
- Integration Capabilities: Python seamlessly integrates with other languages and tools, enhancing flexibility.
---
3. Fundamental Concepts of Machine Learning
Before diving into coding, it’s crucial to grasp some fundamental machine-learning concepts:
- Data Preprocessing: Cleaning and transforming raw data into a suitable format for analysis.
- Feature Engineering: Selecting and creating features that improve the performance of machine learning models.
- Model Training: Teaching the algorithm to make predictions based on data.
- Evaluation Metrics: Assessing the performance of models using metrics like accuracy, precision, recall, and F1-score.
- Overfitting and Underfitting: Balancing model complexity to ensure it generalizes well to new data.
A solid understanding of these concepts will provide a strong foundation for your machine-learning projects.
---
4. Essential Python Libraries for Machine Learning
Python offers a plethora of libraries that simplify machine-learning tasks. Here are some of the most essential ones:
- NumPy: Provides support for large, multi-dimensional arrays and matrices and a collection of mathematical functions to operate on them.
- Pandas: Offers data manipulation and analysis tools, making it easier to handle structured data.
- Scikit-learn: A powerful library for machine learning featuring algorithms for classification, regression, clustering, and more.
- Matplotlib & Seaborn: Visualization libraries that help create informative graphs and plots.
- TensorFlow & Keras: Libraries for deep learning, enabling the creation of complex neural networks.
These libraries form the backbone of most machine-learning projects and are invaluable for beginners and seasoned practitioners.
---
5. Getting Started: Setting Up Your Python Environment
To begin your machine learning journey with Python, you need to set up your development environment. Here’s how:
1. Install Python: Download and install the latest version of Python from the
2. Anaconda Distribution: Consider installing Anaconda, a popular Python distribution that comes pre-packaged with essential data science libraries.
3. Integrated Development Environment (IDE): Choose an IDE that suits your preferences. PyCharm is an excellent choice for Python development, offering powerful features and a user-friendly interface.
4. Create Virtual Environments: Use virtual environments to manage dependencies and organize your projects. Anaconda simplifies this process with built-in tools.
Setting up your environment correctly ensures a smooth and efficient workflow as you develop machine learning models.
---
6. Simple Machine Learning Projects for Beginners
Hands-on projects are the best way to reinforce your learning. Here are some beginner-friendly machine-learning projects to get you started:
- Predicting Housing Prices: Use regression algorithms to predict the prices of houses based on various features.
- Image Classification: Classify images into categories using convolutional neural networks (CNNs).
- Sentiment Analysis: Analyze text data to determine the sentiment expressed, such as positive or negative reviews.
- Customer Segmentation: Group customers based on purchasing behavior using clustering algorithms.
- Spam Detection: Develop a model to identify and filter out spam emails.
Each project introduces different aspects of machine learning, helping you build a well-rounded skill set.
---
7. Recommended Books and Courses
Enhance your learning with these highly recommended resources:
Books:
- *Hands-On Machine Learning with Scikit-Learn, Keras, and TensorFlow* by Aurélien Géron
An in-depth guide that covers practical machine learning techniques using popular Python libraries. [Buy on Amazon]
Courses:
- *Machine Learning by Andrew Ng* on Coursera
A foundational course that introduces machine learning concepts and algorithms. [Enroll on Coursera](https://www.coursera.org/learn/machine-learning)
These resources provide structured learning paths and practical exercises to solidify your understanding of machine learning with Python.
---
8. Conclusion
Embarking on a machine learning journey with Python opens up possibilities in data analysis, predictive modeling, and intelligent decision-making. You can build a strong foundation in machine learning by understanding fundamental concepts, leveraging powerful Python libraries, and engaging in hands-on projects. Additionally, utilizing recommended resources like books, courses, and programming platforms will accelerate your learning and proficiency.
Remember, the key to mastering machine learning is continuous practice and staying updated with the latest advancements. Start with simple projects, gradually tackle more complex problems, and don’t hesitate to seek help from the vibrant Python and machine learning communities.
Start your machine learning journey today with Python and unlock the potential to transform data into actionable insights!
---
*Disclaimer: This post contains affiliate links, meaning we may earn a commission if you click a link and make a purchase at no additional cost. We only recommend products and services we believe will add value to our readers.*
Comments
Please log in to leave a comment.
No comments yet.